Analytics Maturity Model: The Ultimate Guide
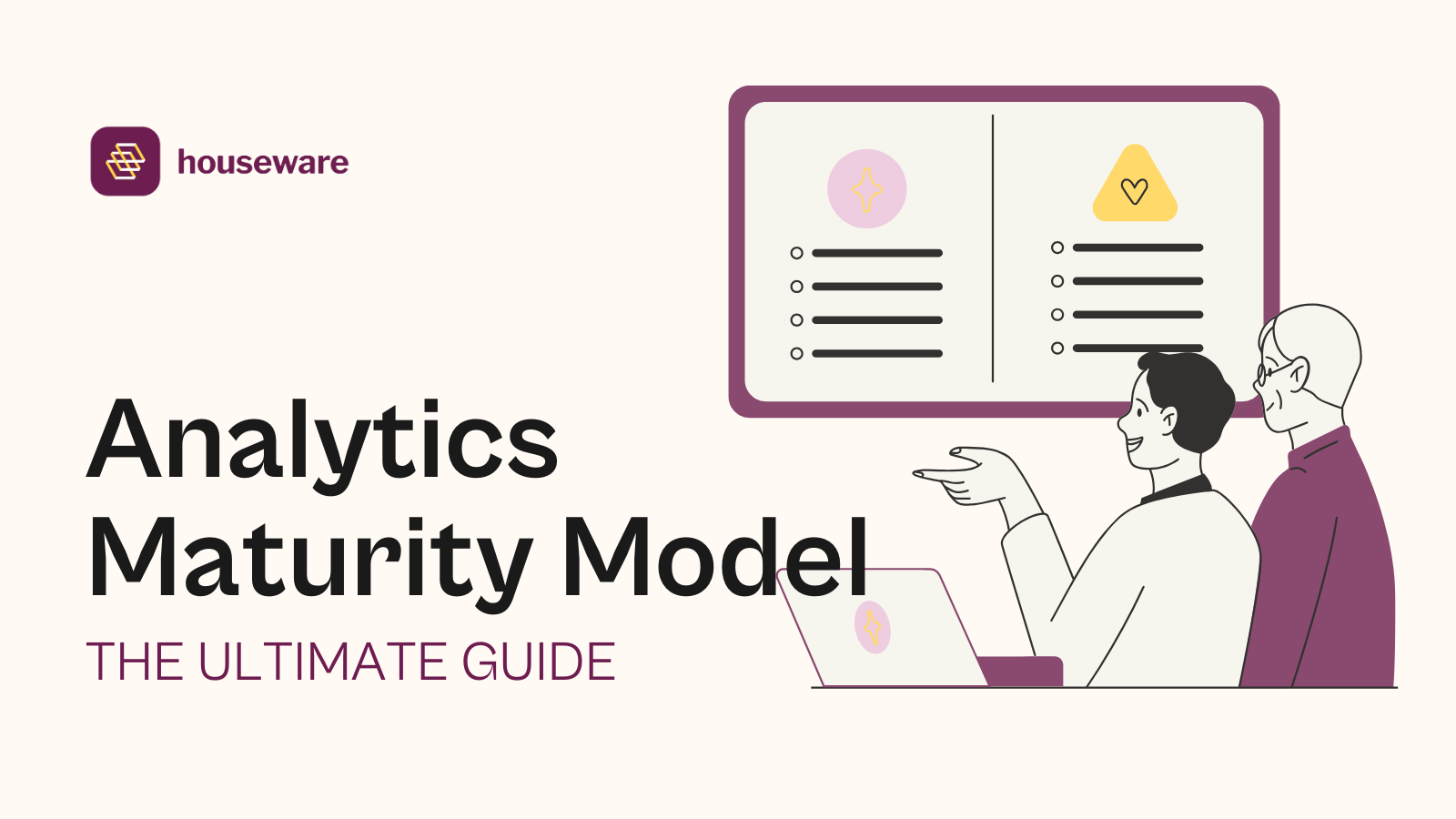
Today, data analytics has become crucial for any organization because of the competitive edge it provides in decision-making. Consequently, having a good analytics framework is essential for streamlined data analysis. However, infrastructure, technology, cost, and other resources can affect your analytics performance. This is where the need for an analytics maturity model arises.
This guide provides detailed information on the different stages of the analytics maturity model and why they are necessary for your data analytics journey.
What is the Analytics Maturity Model?
The Analytics Maturity Model is a framework used by organizations to assess and improve their analytical capabilities. The analytics maturity model helps you determine your current position in the data analysis journey and paves the path for your progress with a clear roadmap.
It typically consists of several stages that represent different levels of sophistication in how data is collected, analyzed, and utilized within the organization. These levels often progress from basic descriptive analytics to more advanced predictive and prescriptive analytics.
Some of the analytics maturity models frequently used by organizations are:
- Analytic Processes Maturity Model (APMM)
- Analytics Maturity Quotient Framework
- Gartner's Maturity Model for Data and Analytics
- Logi Analytics Maturity Model
- Online Analytics Maturity Model (OAMM)
- Blast Analytics Maturity Assessment Framework
- Data Analytics Maturity Model for Associations (DAMM)
- DELTA Plus Model
- SAS Analytic Maturity Scorecard
- TDWI Analytics Maturity Model
- Web Analytics Maturity Model (WAMM)
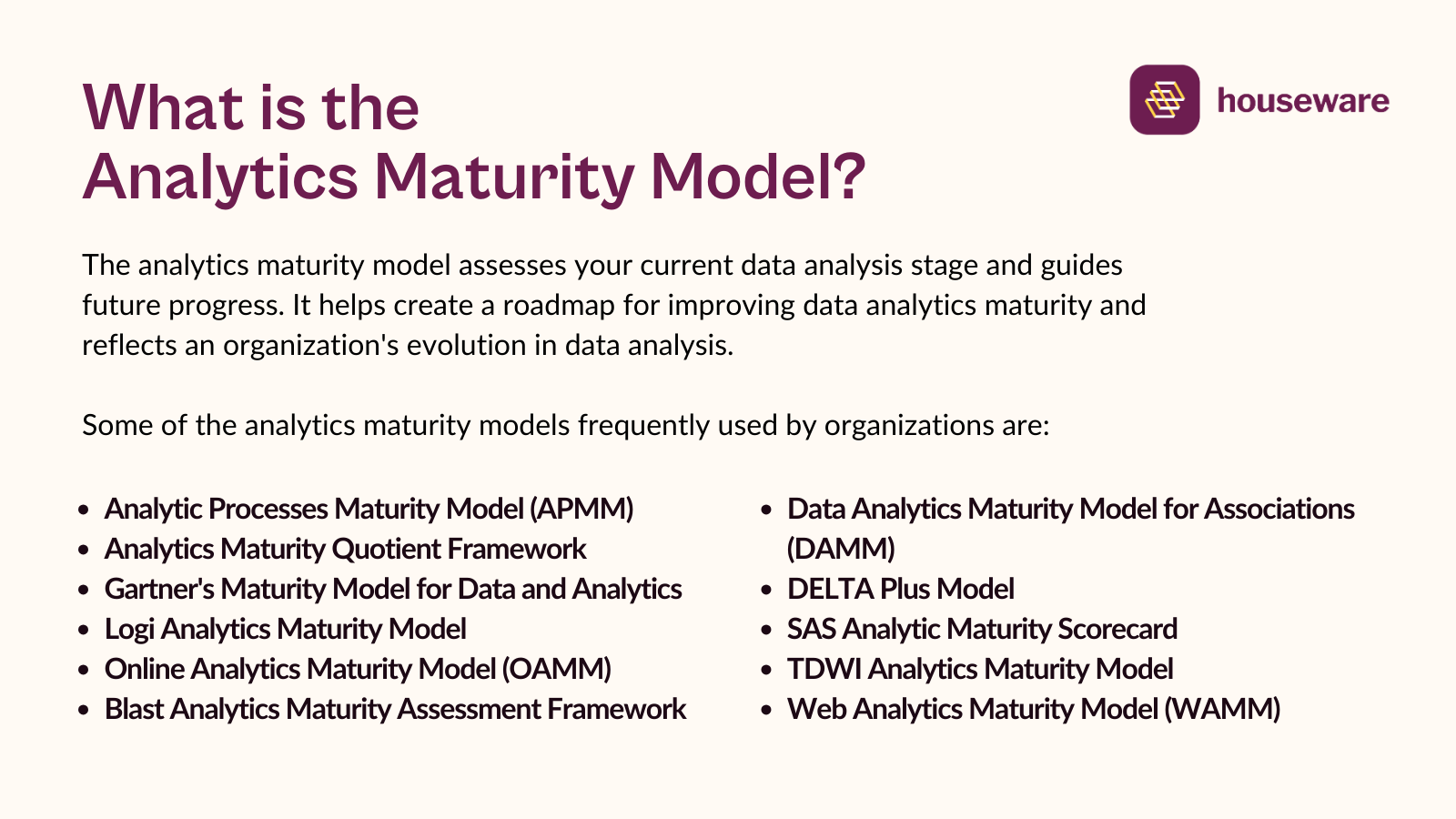
Here, we are going to discuss Gartner’s maturity model in detail.
What are the Stages of an Analytics Maturity Model?
Any organization goes through six prominent stages of analytics maturity. They are:
- Ground or Foundational Stage
- Descriptive Stage
- Diagnostics Stage
- Predictive Stage
- Prescriptive Stage
- Cognitive Stage
Let's briefly examine each of them. Except for foundational and cognitive stages, the rest are also part of Gartner’s Maturity Model.
.png)
- Foundational Stage: The Foundational Stage in the Analytics Maturity Model represents the initial level of maturity where organizations begin to establish the basic infrastructure and capabilities necessary for effective data analytics. The questions you must ask during this stage are: "What data do I need to make my business decisions better?" and "How and where can I obtain relevant data?”. Here you will need to think through and make decisions on the data collection, management, governance, tech stack, and stakeholder management.
- Descriptive Stage: Many organizations find themselves in this stage where you can get answers to simple questions like “What happened?”, “What are my sales figures?”, and “How much revenue is generated?”. You can use BI tools to find accurate answers. This is where you have basic reporting, ad-hoc analysis, and limited predictive power.
- Diagnostic Stage: The diagnostic stage is built on the descriptive stage. It goes deeper into understanding what outcomes to expect from the analysis and the reasons behind some correlations and causations in the data variables. It usually involves using statistical techniques like bi-variate or multivariate analysis to infer patterns. Here, you can seek answers to questions like, “Why did the event occur?”. Exploring past data helps you draw insights about the present.
- Predictive Stage: Here, organizations analyze “What will happen, why, and when?” based on the insights gathered in the previous two stages. The predictive stage helps you analyze the future trends in your sales and growth. You will find yourself using feature engineering to build machine learning models for obtaining better predictive insights.
- Prescriptive Stage: Prescriptive stage is the enhanced stage of predictive analytics, where you ask questions like “What should happen?” and “What should the company do?”. It intends to understand the company's future trends compared to the market ecosystem and its competitors. You may use machine learning and deep learning tools to draw insights from your data.
- Cognitive Stage: It is the last stage of the analytics maturity model. Here, companies try to draw real-time insights from high-scale data using machine learning and natural language processing. This is a completely automated stage where AI is used to get deeper insights into significant data patterns. To reach this stage, your organization needs discipline to go through the previous stages effectively.
Need for an Analytics Maturity Model
A data analytics maturity model is important for understanding the domain, industry, and market that you function in. Here are some of the prominent reasons why you need an advanced analytics maturity model:
- Assessment and benchmarking: To get clarity on the current status of data analytics and thereby make informed business decisions.
- Goal Setting and Planning: To document the progress and success of your approach towards practical data analysis.
- Continuous Improvement: For assessing the practices adopted by your organization and creating a roadmap for progress.
- Resource Allocation: To improve productivity and performance at different departmental levels by allocating resources correctly.
- Competitive advantage: To adopt newer technologies to remain abreast of the latest trends for obtaining competitive advantage.
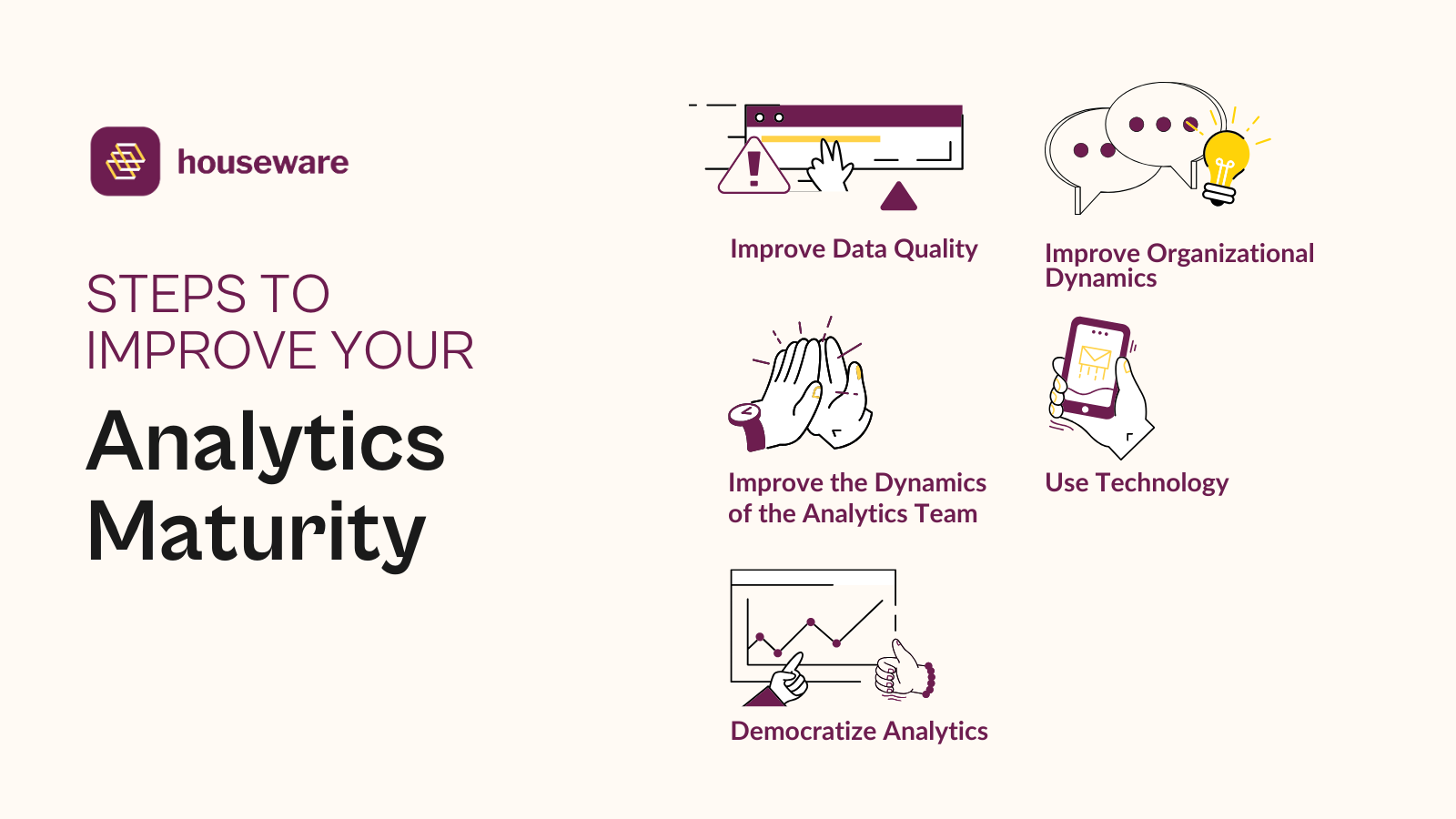
The stages of the analytics maturity model are interdependent. Evaluating your organization against each stage is important for saving time and resources.
Challenges of Analytics Maturity Model Assessment
The journey to analytics maturity is not linear. It is a compound process in which you reach a new stage on the foundation of the previous one. For example, if you do not know “what happened?” in the diagnostics stage, you will not know “what will happen?” in the predictive stage. Some of the challenges that you will usually face in analytics maturity model assessment are:
- Adopting a one-size-fits-all approach may not be efficient for different departments of your company. Each will have its own nuances. So, you should try to do a uniform but department-specific analytics maturity assessment.
- Do not try to jump to the cognitive stage directly. It will not resolve the root cause of problems in your data analytics. Instead, focus on incremental progress.
- Most of the organizations follow a traditional way of doing data analytics. You can try to encourage your employees to adapt and learn technical skills to enhance your data analytics processes.
Steps to Improve Your Analytics Maturity
Once you identify challenges or loopholes in your data analytics, you can follow these steps to improve them:
- Improve Data Quality: To do this, you must centralize all your data at a single location, like a data warehouse or a data lake. You can use automated data migration tools to create a single source of truth. This speeds up your reporting and analytics process.
- Improve Organizational Dynamics: Invest in enhancing your employees' technological skills and know-how. Also, ensure that there is access to IT support whenever any issue arises regarding data integrity or security.
- Improve the Dynamics of the Analytics Team: Clarify your analytics goals with all your teams. Incorporate feedback from stakeholders, employees, and customers to develop a blueprint for optimized analytics performance.
- Use Technology: Having the right IT infrastructure makes a big difference in attaining analytics maturity. Invest wisely in technology such as machine learning, AI, and data science to leverage its benefits. Look out for tools, platforms, and developers who can help you achieve your goals within the set budgets.
- Democratize Analytics: Provide access to your teams to carry out self-serving analytics, which will create autonomy in decision-making processes. Reduce the dependency on technical teams and data scientists for gathering insights by training your interdependent teams with the necessary analytics skills.
Houseware: An Analytics Platform to Enhance Your Analytics Maturity
Houseware is a product analytics platform that helps you improve your data analytics process. You can connect to customer data platforms and data warehouses to get insights into customer metrics, trends, and demand patterns. Several features of Houseware make it a trusted analytics platform. Some of these are:
Trends: The trends feature of Houseware allows you to examine the metrics and composition of your data. You can easily configure the exact trend chart by selecting any event of your choice. It helps you identify patterns in your data through descriptive, diagnostic, and predictive analytics.
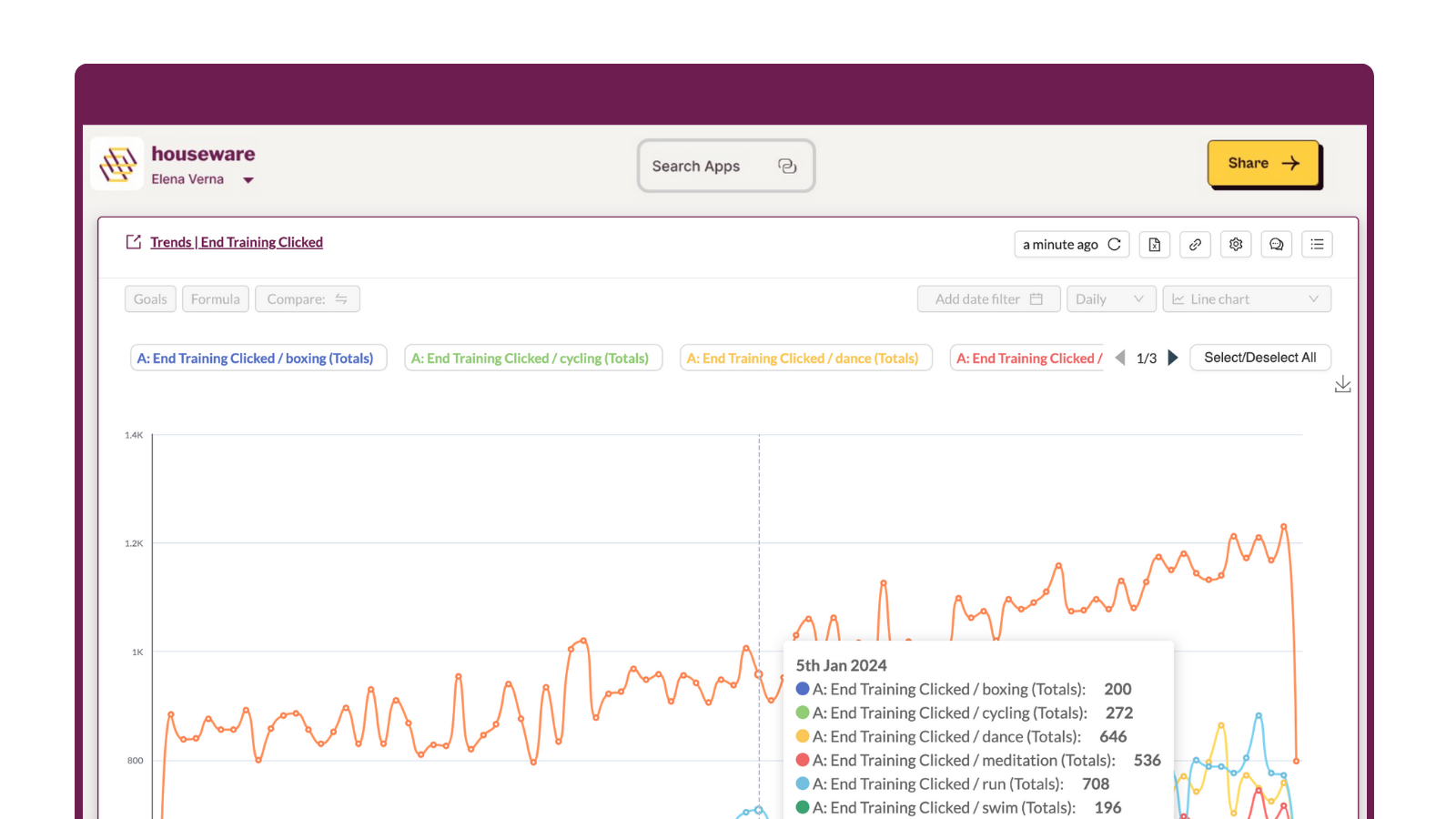
Stickiness: This Houseware feature helps you understand customers' behavior toward your product. It measures the frequency with which a user engages with your product. High stickiness implies that your product is providing value to the customer.
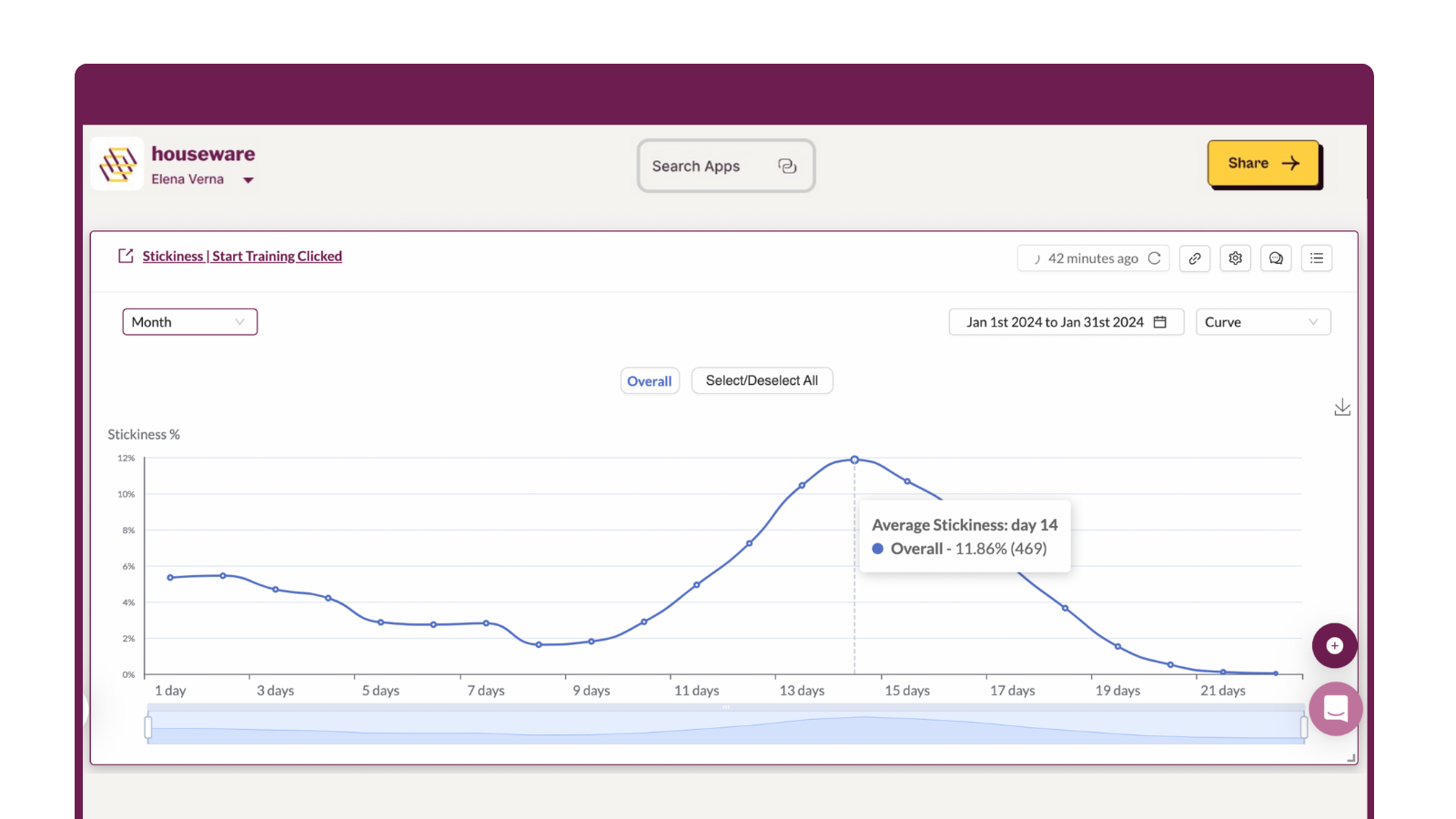
Flows: Flow charts are visuals of paths users take frequently to interact with your product. Monitoring and analyzing these paths helps you gain information about how users engage with your product. This is helpful at the prescriptive and cognitive stages of the analytics maturity model.

Conclusion
This article provides a comprehensive guide on the role of the analytics maturity model in data analytics. It provides insights into the various stages of the analytics maturity model and the challenges associated with them. You should perform an analytics maturity assessment of your data to evaluate and improve your current position on the scale of analytics maturity.

Related Blogs
